Negative results. Statistically insignificant outcomes. Why do we rarely see published papers like this? Why does everything have to have a statistically viable effect or difference? At first glance, it makes total sense. Of COURSE we want to be able to see if people like burrito A better than burrito B and use probability and statistics to determine if it was just a fluke or if it was statistically better. BUT–what if burrito A and burrito B are exactly the same? What if no one actually cares which burrito they enjoy? Isn’t that information just as valuable as if burrito A was better than B?
For those of you familiar with statistics and probability, you can skip the next few paragraphs.
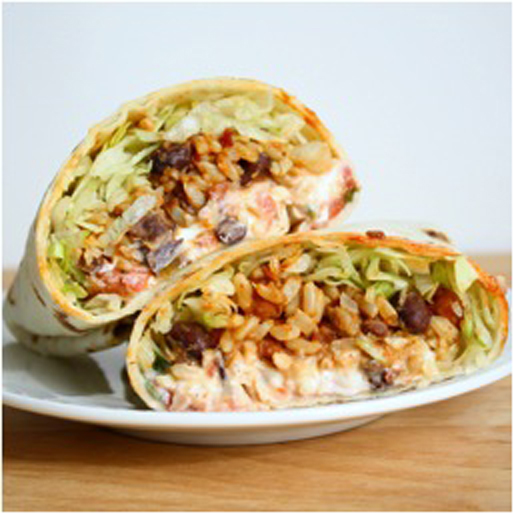
I don’t know why I like using burritos in statistics, but I just do.
Who doesn’t like an awesome, loaded burrito? NO ONE.
——–
Crash course in statistical probability
Statistics are not as scary as people think. I tutor statistics here at Seattle U and I know that the math can be daunting, but if broken down into bite-sized chunks of concept as it relates to math, it’s a whole lot easier to understand logically. For example (bear with me, this statistics lesson has a point), a sign test can determine if the number of people who like burrito A is actually more than burrito B by using probability. Think of it this way: if burrito A was heads of a quarter and burrito B was tails, what number of heads would I need to get in order to have a totally improbable amount compared to tails?
In statistics we use something called an alpha. An alpha is the arbitrarily chosen threshold for if something is “statistically significant” or not. Usually in psychology we use an alpha of .05 or .01, which means that the result in question should be 5% or 1% chance that it was a fluke (or a 95% or 99% chance of it NOT being due to chance).
In the case with the burritos, we need to calculate the probability of flipping a certain number of heads to tails ratio and determine if that is due to chance or not. If getting 9 heads out of 10 flips happens, we know for sure that that’s statistically significant because each flip is a 50/50 chance. If each flip has a 50% chance of going to heads and we extrapolate that out using basic probability, the total probability for getting 9 heads out of 10 flips is 0.00977*, which is much less than 0.05, our predetermined alpha.
MAGIC! Now we know that 9/10 heads is statistically significant and that the chance for each trial is likely weighed in the favor of heads. If applied back to the burrito example, this basically says that there was NOT a 50/50 chance of people liking burrito A over burrito B and that people are more likely to prefer burrito A.
The same principle applies to the more complicated statistical tests.
——-
The main problem with a lot of science today is that no one reports the stuff that ISN’T significant. Why should we care about insignificant results? Because they can tell us information that is just as valuable as significant ones. For example, if someone wanted to see if antidepressants would be good for weight loss, an insignificant result would say that antidepressants are NOT good for weight loss. Seems like pretty good information, right? Now what if that trial was never published in a journal and someone else came along and wanted to test it out? They would have never seen that result and wouldn’t know what people had already done in that field.
Sharing negative results is like warning someone about a bad professor. They could figure it out themselves, but giving them a little guidance going in could have helped them better in the long run by telling them how to study or what to expect. Negative results may not be as flashy or popular, but they also tell us what could be wrong with the study’s methods or sample, or any number of other factors that may influence the results. Sharing the complete methods and information with others enables scientists to make better informed decisions and to build on a foundation of knowledge. Without negative results, we wouldn’t see a large portion of that foundation.
——
*The math is shown below.
N = 10 tosses
p=0.5 (1/2 chance of each outcome, heads or tails)
x=9 (number of heads)
10 x (0.5)^9 x (1-0.5) = 10 x (0.5)^10 = 0.00977
Also, here’s a recipe for a tasty burrito.
http://abitchinkitchen.blogspot.com/2011/10/spicy-bean-and-rice-burritos.html